2. Building analysis pipelines with targets
1 The targets
package
1.1 What does the targets
package do?
The {targets}
package is a framework for building data processing, analysis and visualization pipelines in R. It helps you structure your code by splitting it onto logical chunks that follow each other in a logical order. {targets}
also manages the intermediate results of each step, so you do not have to do it manually. It also minimizes the time it takes to update the results if any step changes, be it some change in the source data, or in the code. {targets}
knows which steps are up-to-date, and which are not, and only runs the steps that are outdated.
Consider the following minimal example:
library(targets)
list(
# 1. Load the mtcars dataset.
tar_target(
name = mtcars_data,
command = data(mtcars)
),
# 2. Compute the mean of the mpg column.
tar_target(
name = mpg_mean,
command = mean(mtcars_data$mpg)
),
# 3. Create a histogram plot of mpg using base R.
tar_target(
name = hist_mpg,
packages = c("ggplot2"),
command = {
ggplot(mtcars_data, aes(x = mpg)) +
geom_histogram(binwidth = 1) +
labs(title = "Histogram of MPG", x = "Miles Per Gallon", y = "Count")
}
) )
This script has three steps. It loads the data, computes a simple statistic, and creates a plot. To run this pipeline with targets
you would need to save this script to the _targets.R
file in the root of the project directory and run targets::tar_make()
in R console.
Reveal code to try the toy targets
example above in R console interactively
To quickly run the pipeline above in R console without setting up targets
properly and creating an R script file, you can use the code below, that can be pasted in to the R console (and it will make the pipeline run in a temporary directory):
library(targets)
tar_dir({
tar_script({
library(targets)
list(
# Load the mtcars dataset.
tar_target(mtcars_data, mtcars),
# Compute the mean of the mpg column.
tar_target(mpg_mean, mean(mtcars_data$mpg)),
# Create a histogram plot of mpg using base R.
# The plot is saved as a PNG file and the filename is returned.
tar_target(plot_mpg, {
png("hist_mpg.png") # Open a PNG device.
hist(mtcars_data$mpg,
breaks = "Sturges",
main = "Histogram of MPG",
xlab = "Miles Per Gallon",
ylab = "Frequency",
col = "lightblue")
dev.off() # Close the device.
"hist_mpg.png" # Return the filename as the target value.
})
)ask = FALSE)
},
# Run all targets defined in the pipeline.
tar_make()
})
You will get output similar to this:
▶ dispatched target mtcars_data0 seconds, 1.225 kilobytes]
● completed target mtcars_data [
▶ dispatched target plot_mpg0.011 seconds, 65 bytes]
● completed target plot_mpg [
▶ dispatched target mpg_mean0 seconds, 52 bytes]
● completed target mpg_mean [0.059 seconds] ▶ ended pipeline [
It would mean that all steps have been executed successfully.
Similarly, if you add tar_visnetwork()
after tar_make()
, you will get a visualization of the pipeline:
library(targets)
tar_dir({
tar_script({
library(targets)
list(
# Load the mtcars dataset.
tar_target(mtcars_data, mtcars),
# Compute the mean of the mpg column.
tar_target(mpg_mean, mean(mtcars_data$mpg)),
# Create a histogram plot of mpg using base R.
# The plot is saved as a PNG file and the filename is returned.
tar_target(plot_mpg, {
png("hist_mpg.png") # Open a PNG device.
hist(mtcars_data$mpg,
breaks = "Sturges",
main = "Histogram of MPG",
xlab = "Miles Per Gallon",
ylab = "Frequency",
col = "lightblue")
dev.off() # Close the device.
"hist_mpg.png" # Return the filename as the target value.
})
)ask = FALSE)
},
# Run all targets defined in the pipeline.
tar_make()
tar_visnetwork()
})
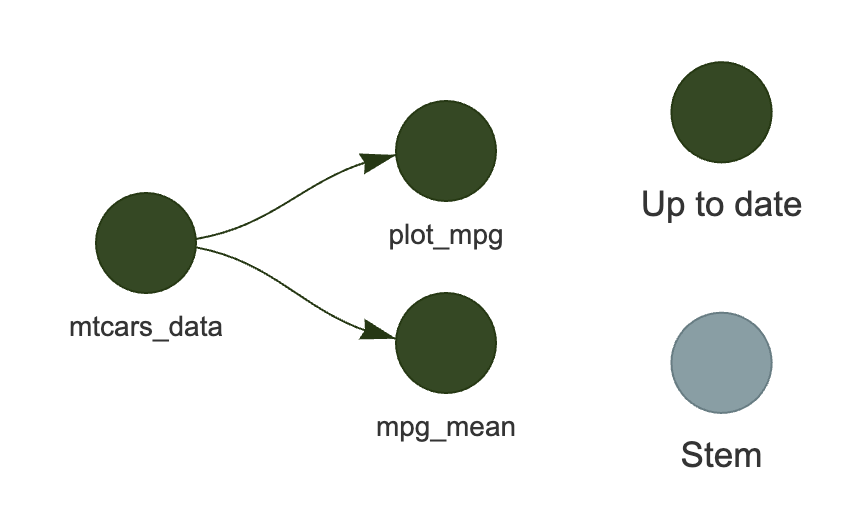
As you can see in Figure 1, {targets}
knows that the summary statistic and the plot depend on the data. If you were to change the first step of the pipeline, then the next time you ask {targets}
to update the pipeline, it would only run steps two and three. If, however, instead of changing the data, you changed the code for the plot (e.g. change the title), then the pipeline would only re-run step three. This way, you do not have to manually keep track of what might have changed in the data or the code, or, in more complex pipelines, in the intermediate data (such as cross-validation folds for machine learning models) - {targets}
will figure it out for you.
2 Exercise
2.1 Goal
The goal of this exercise is to use the project folder that you have set up in the previous exercise with {renv}
to create a simple analysis pipeline using {targets}
.
If you have not successfully completed the previous exercise, you can start the current one by downloading the repository https://github.com/e-kotov/2025-mpidr-workflows-reference-01 and extracting it to your computer.
2.2 Instructions
2.3 Open the project folder in your editor
Open either your own project, or our reference project snapshot form the Note above.
If you open your own project, you should see in the R console:
- Project 'path/on/your/computer/to/your/project' loaded. [renv 1.1.1]
If you open our reference project, you should see in the R console:
OK- Installing renv ... OK
- Project 'path/on/your/computer/2025-mpidr-workflows-reference-01' loaded. [renv 1.1.1]
- One or more packages recorded in the lockfile are not installed.
- Use `renv::status()` for more details.
The reason you will see that the packages are not installed, is because the library of R packages is specific to an operating system and is considered as a disposable storage. By default, it is not pushed to GitHub. Therefore, when you copy such project from GitHub, you don’t have the packages, but you have all the files to restore the packages as required by the renv.lock
file.
2.4 Restore (install) the R packages in the project
2.4.1 In your own project
In your own project, you need to run:
::status() renv
This is to make sure that all the packages are installed in the project. If any packages are still missing, see the step below.
2.4.2 In our reference project
If you are working in our reference project from https://github.com/e-kotov/2025-mpidr-workflows-reference-01, you need to run:
::restore() renv
After you confirm, you should see the package installation process.
Once the installation finishes, to make sure you have successfully installed all packages, run:
::status() renv
You should get something like this:
-- the project is in a consistent state. No issues found
2.5 Setup the {targets}
pipeline
You could of course create a targets
pipeline manually, but there is a handy R function to initialize a template for you:
::use_targets() targets
Agree to that and {renv}
will take over again to install a new package. Don’t forget to add it to the renv.lock
file with renv::snapshot()
later.
Once this is done, you should get a new file _targets.R
in the root of your project folder.
2.6 Explore the sample pipeline
Before editing the _targets.R
file, explore the pipeline that is already defined in the file.
First, run this to see the interactive graph of the pipeline (you will need to agree to install visNetwork
package when you run this for the first time, run the command again if you still don’t see the visualisation in the viewer):
::tar_visnetwork() targets
You will see that all steps are currently outdated.
Now you can runn all the steps in the pipeline:
::tar_make() targets
Notice that you a new folder called _targets
is created in the project folder. This is where the output of the pipeline will be stored as well as some metadata.
If you run targets::tar_visnetwork()
again, you will find all steps are now up-to-date.
Now you can inspect in the R
console the results of each step. You do not need to think where each output is saved, as all outputs are always stored in the _targets
folder and are accessible by their name. For example:
<- targets::tar_read(data)
tmp_object print(tmp_object)
rm(tmp_object)
This will read the data
object that is the output of the first step of the example pipeline and print it to the console. Finally, we also remove this object from the workspace to keep it clean.
You may also load the object data
with its name directly into the workspace with:
::tar_load(data) targets
ls()
1] "data" [
Now clean the workspace/environment:
rm(list = ls())
To delete saved outputs of all or certain (see the documentation) steps, run:
::tar_destroy() targets
To delete the saved results of steps that you might have deleted and will not use anymore, you could run:
::tar_prune() targets
2.7 Adjust targets
settings
Feel free to remove most of the comments in the example _targets.R
file, especially the ones regarding the targets options. We recommend you do set the options as follows:
tar_option_set(
format = "qs"
)
The qs
/qs2
format is a faster and more space efficient alternative to rds
format. You can read more about it in the qs2
documentation. This will be the format that targets
uses to save the output of each step of the pipeline, unless you specify format = 'file'
, or set it to something else in the specicfic tar_target()
step.
You might also notice that we suggest to remove the packages
option from tar_option_set()
that was originally there. This is because it is much safer to specify the packages you need in each tar_target()
step individually. This might seem like a lot of work, but it it has many advatanges:
- Each step runs much faster, as unnecessary packages are not loaded.
- You can prevent potential package conflicts. In big projects with hundreds of packages, some packages may not work well when loaded simultaneously (which would happen if you specify the
packages
option globally for all steps). - It is much easier to debug, as you can see which packages are loaded at each step.
For more targets
options, you can see the documentation for tar_option_set()
.
2.8 Analysis step 1 - Get the data
See the code in Jonas’s repository at https://github.com/jschoeley/openscience25/tree/main/layer2-communal/example_2-1.
Let us try to implement the steps from his code in a targets
pipeline. We will guide you through the first pipeline step together.
2.8.1 Wrap data download step into a function
We first need to implement the data download step found in https://github.com/jschoeley/openscience25/blob/main/layer2-communal/example_2-1/code/10-download_input_data_from_zenodo.R.
We will keep all functions that implement the steps in a separate folder called R
. Create this folder manually and create a file called 10-download_input_data_from_zenodo.R
in it. Copy the code from Jonas’s repository and save it in this new file, but convert it to a function that we could call from the targets
pipeline. Compared to Jonas’s original code, this function needs to make sure that the folder for the file downloads exists and also return the paths to the downloaded data files. See the suggested function below:
# Download analysis input data from Zenodo
<- function(
download_files_from_zenodo data_folder = "data"
) {# make sure the data folder exists
if (!dir.exists(data_folder)) {
dir.create(data_folder, recursive = TRUE)
}
# download the files
download.file(
url = 'https://zenodo.org/records/15033155/files/10-euro_education.csv?download=1',
destfile = paste0(data_folder, "/10-euro_education.csv")
)
download.file(
url = 'https://zenodo.org/records/15033155/files/10-euro_sectors.csv?download=1',
destfile = paste0(data_folder, "/10-euro_sectors.csv")
)
download.file(
url = 'https://zenodo.org/records/15033155/files/10-euro_geo_nuts2.rds?download=1',
destfile = paste0(data_folder, "/10-euro_geo_nuts2.rds")
)
# find the downloaded files
<- list.files("data", full.names = TRUE)
data_from_zenodo
# return the list of files
return(data_from_zenodo)
}
Compare this to the original code from Jonas’s repository at https://github.com/jschoeley/openscience25/blob/main/layer2-communal/example_2-1/code/10-download_input_data_from_zenodo.R.
Now, to implement this first step into the pipeline, let us find the list of steps in the end of the _targets.R
file, it’s the one where all the steps are listed, each starting with tar_target()
.
Remove all the example steps and insert your own:
list(
# download files from zenodo
tar_target(
name = data_from_zenodo,
command = download_files_from_zenodo(
data_folder = "data"
),format = "file"
) )
Notice that we have added format = "file"
to our first step. This means that targets
should expect not a generic R
object like a data.frame
, list
, or vector
as on output of the download_files_from_zenodo()
function, but a vector of files. This is useful for actions such as file downloads, as targets
will be checking on it’s own if all expected files are actually downloaded.
The final step is to inform targets
that we want to run the pipeline by executing the targets::tar_make()
.
2.8.2 Download files by executing the pipeline
Now you can run the pipeline by executing:
::tar_make() targets
You should get something like this:
▶ dispatched target data_from_zenodo'https://zenodo.org/records/15033155/files/10-euro_education.csv?download=1'
trying URL 'text/plain; charset=utf-8' length 11150 bytes (10 KB)
Content type ==================================================
10 KB
downloaded
'https://zenodo.org/records/15033155/files/10-euro_sectors.csv?download=1'
trying URL 'text/plain; charset=utf-8' length 20626 bytes (20 KB)
Content type ================================
20 KB
downloaded
'https://zenodo.org/records/15033155/files/10-euro_geo_nuts2.rds?download=1'
trying URL 'application/octet-stream' length 261344 bytes (255 KB)
Content type =======
255 KB
downloaded
0.894 seconds, 293.12 kilobytes]
● completed target data_from_zenodo [0.94 seconds] ▶ ended pipeline [
The pipeline downloaded the files from Zenodo and will save them into the data
folder. You can now use the downloaded files in the next steps of the pipeline.
Make an interactive graph of the pipeline:
::tar_visnetwork() targets
You should see a network similar to the one in Figure 2.
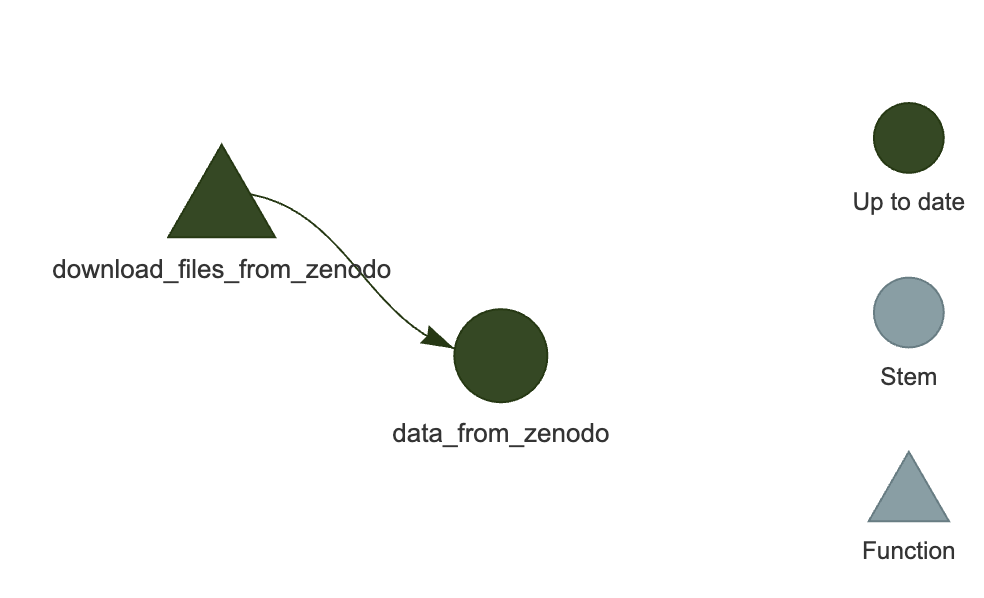
One thing to try, is to manually delete any one of the downloaded files and run the targets::tar_visnetwork()
function. You will then see that one of the steps is outdated, as targets
knows from the first run that it needs to keep track of files and it has noted the file signatures.
2.8.3 Add data folder to .gitignore
As the data is preserved at Zenodo, and as it is bad practice to store data in the git repository, we should add the data
folder to the .gitignore
file. You can do this by executing the following command in the R
console:
::use_git_ignore("data") usethis
This will create a new .gitignore
file in the root of the project (in case it did not exist) and add the data
folder there. Now git
tools will not prompt you to commit the data
folder and it will never be pushed to GitHub or other remote repository you are using.
2.9 Analysis step 2 - Create background map
Now let us add a new step that creates background map that will be re-used in all of the plots me can create following the code in Jonas’s repository. We will look at the code in https://github.com/jschoeley/openscience25/blob/main/layer2-communal/example_2-1/code/20-create_european_backgroundmap.R and adjust it to be a targets
step executed by tar_target()
.
Because the code there uses _config.yaml
to customize the plots, you will need to download it and put into your project, e.g. into config
folder.
We will also break down the code into steps, as it makes sense to isolate the code for downloading the data from Natural Earth into a separate function.
2.9.0.1 Step 2.1 Download Natural Earth data
Look at the corresponding lines in Jonas’s code:
# Download Eurasian geodata ---------------------------------------
<-
eura_sf # download geospatial data for European, Asian and African countries
ne_countries(continent = c('europe', 'asia', 'africa'),
returnclass = 'sf', scale = 10) %>%
# project to crs suitable for Europe
st_transform(crs = config$crs) %>%
# merge into single polygon
st_union(by_feature = FALSE) %>%
# crop to Europe
st_crop(xmin = config$eurocrop$xmin,
xmax = config$eurocrop$xmax,
ymin = config$eurocrop$ymin,
ymax = config$eurocrop$ymax)
We will rewrite it as a following function:
# Download Eurasian geodata ---------------------------------------
<- function(
download_ne_data
crs, xmin, xmax, ymin, ymax
) {<-
eura_sf # download geospatial data for European, Asian and African countries
::ne_countries(
rnaturalearthcontinent = c('europe', 'asia', 'africa'),
returnclass = 'sf',
scale = 10
|>
) # project to crs suitable for Europe
::st_transform(crs = crs) |>
sf# merge into single polygon
::st_union(by_feature = FALSE) |>
sf# crop to Europe
::st_crop(xmin = xmin, xmax = xmax, ymin = ymin, ymax = ymax)
sf
return(eura_sf)
}
As you can see, we got rid of the references to yaml
file, which is one of the ways to approach it. Any preferences previously set in yaml file are now set in the function parameters.
We also prefixed each function call with a package name. We do this to be more aware of which packages we use in each function to minimize the potential for conflicts.
In the list of targets
steps we will add the call as follows:
tar_target(
name = eura_sf,
packages = c("rnaturalearth", "sf"),
command = download_ne_data(
crs = 3035,
xmin = 25.0e+5,
xmax = 75.0e+5,
ymin = 13.5e+5,
ymax = 54.5e+5
) )
Note that:
- We specified the
packages
argument for an individual target step. - We did not specify the
format = "file"
, so by default it will beqs2
format, as specified above intar_option_set()
.
As you might have noticed, we set the name of the targets
step as eura_sf
, and we also return an object called eura_sf
from the download_ne_data()
function. These names do NOT have to match, as in larger projects functions may be reused multiple times and they may have more generic uses and therefore return different output to different targets based on their input parameters. In this case, we are just naming the objects in the same way for convenience.
Test the targets
pipeline by running it and making an interactive graph:
::tar_make()
targets::tar_visnetwork() targets
You should see a network similar to the one in Figure 3.
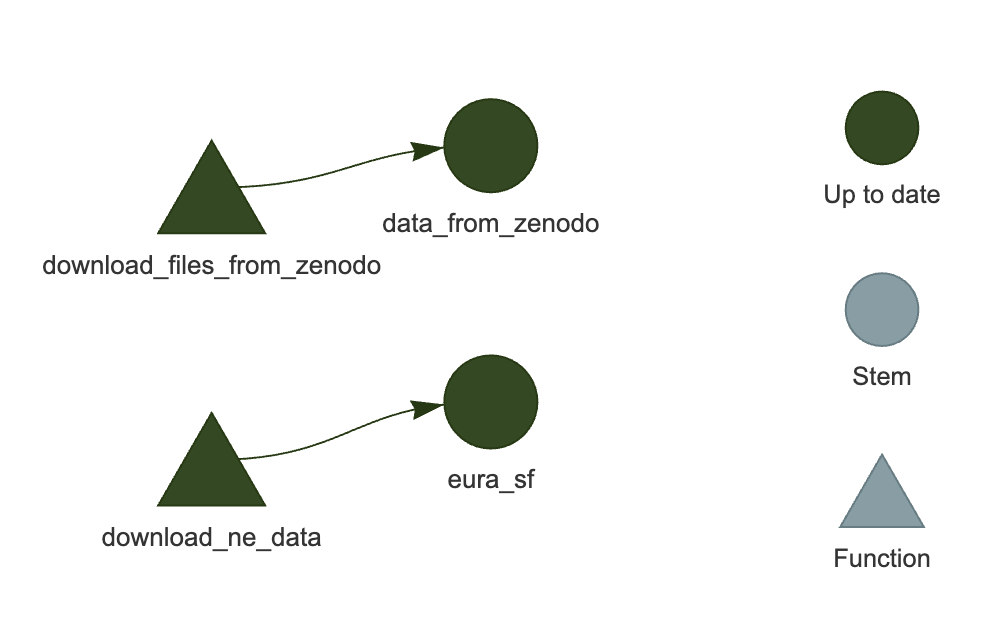
2.10 Step 2.2 Wrap background map creation step into a function
Create a file 20-create_european_backgroundmap.R
in R
subfolder of your project.
Look at the first few lines in Jonas’s code, just after the library()
calls (which we will look at later).
Original lines:
# input and output paths
<- list()
paths $input <- list(
pathsconfig = './code/_config.yaml'
)$output <- list(
pathseuro_basemap.rds = './data/20-euro_basemap.rds'
)
# global configuration
<- read_yaml(paths$input$config) config
We do not nede to use any of these lines anymore. Previously we had to specify where to save the base map in paths$output
. With targets
, we do not need to manually keep track where we save intermediate results and wether or not they are up to date. Instead of saving the file at the path specified in paths$output
, we will just return an R
object from the function called by a particular targets
step and it will be stored automatically for us in _targets/objects
folder. We do not need the _config.yaml
here anymore, as it did not contain any variables that would affect the basemap creation.
We only need the lines that create a ggplot2
plot object with the basemap:
# Draw a basemap of Europe ----------------------------------------
<-
euro_basemap ggplot(eura_sf) +
geom_sf(
aes(geometry = geometry),
color = NA, fill = 'grey90'
+
) coord_sf(expand = FALSE, datum = NA) +
theme_void()
And we rewrite these as a function:
# Draw a basemap of Europe ----------------------------------------
<- function(
create_european_backgroundmap
eura_sf
) {# eura_sf <- targets::tar_read(eura_sf) # for debug
<-
euro_basemap ::ggplot(eura_sf) +
ggplot2::geom_sf(
ggplot2aes(geometry = geometry),
color = NA,
fill = 'grey90'
+
) ::coord_sf(expand = FALSE, datum = NA) +
ggplot2::theme_void()
ggplot2
return(euro_basemap)
}
Notice that we pass eura_sf
object that we created with targets
step that executed download_ne_data()
and we return the ggplot2
object euro_basemap
that should be reused in the next steps for creating the maps.
We also added explicit ggplot2::
prefixes to relevant functions. This is optional, as we will specify that we need ggplot2
package for this function in the targets
pipeline, but this also helps to debug the function quickly.
To run the code in this function manually, you can use the commented out line eura_sf <- targets::tar_read(eura_sf)
to quickly load the eura_sf
object (assuming you have previously ran the targets
pipeline and it is saved in the targets/ojects
storage).
Now let us add a targets
step to the pipeline:
tar_target(
name = euro_basemap,
packages = c("ggplot2"),
command = create_european_backgroundmap(
eura_sf = eura_sf
) )
Test the targets pipeline by running it and making an interactive graph:
::tar_make()
targets::tar_visnetwork() targets
You should see a network similar to the one in Figure 4.
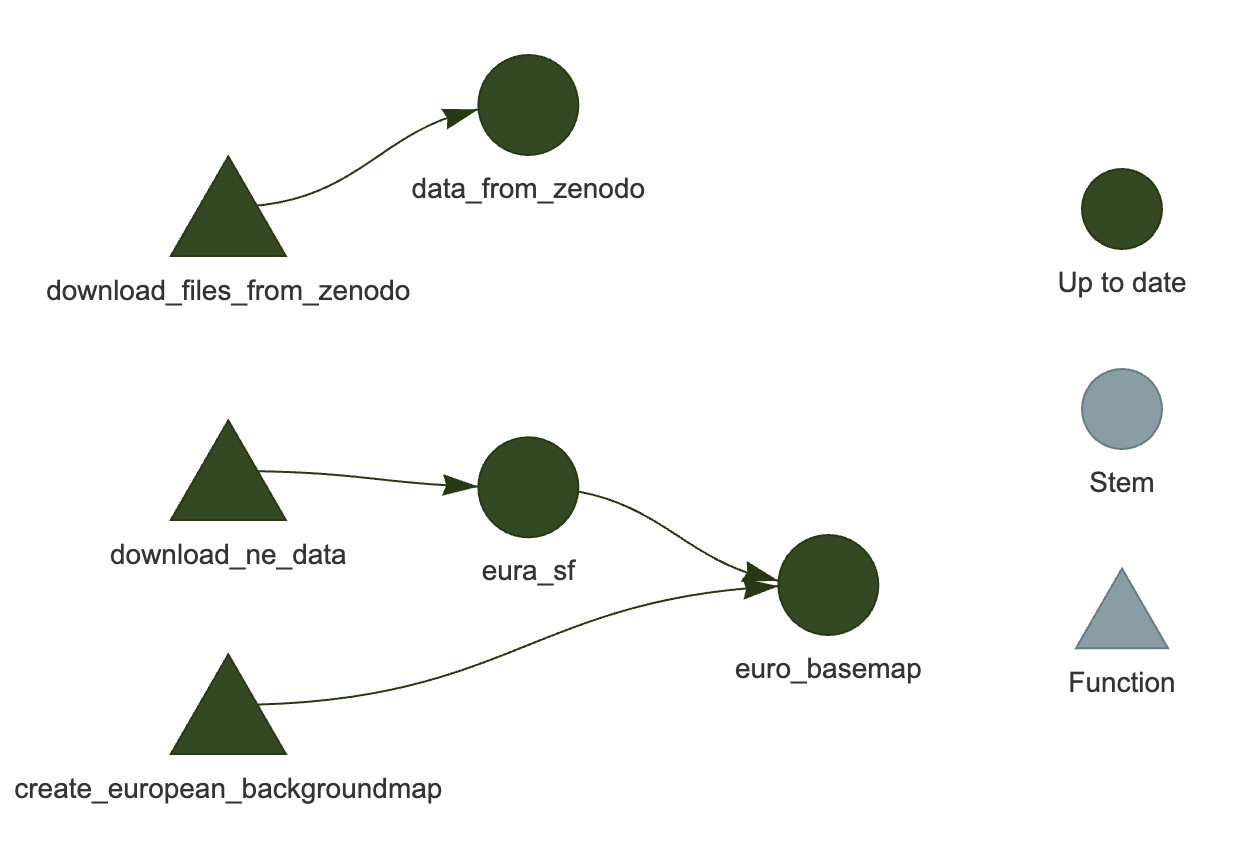
Now if you run targets::tar_read(euro_basemap)
, you should get a plot of the basemap (see Figure 5):
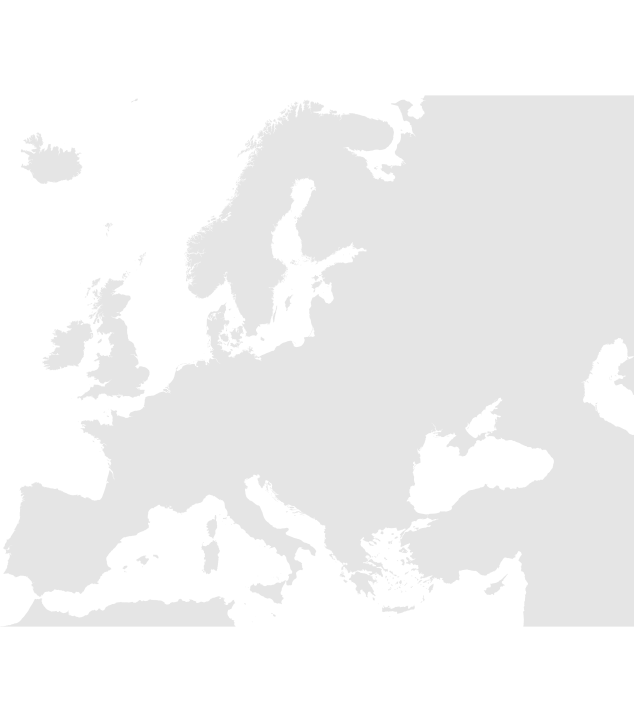
targets::tar_read(euro_basemap)
2.11 Next steps
Now you it is up to you to adapt the code from one of the R scripts that create maps (30-plot_figure_1.R
, 31-plot_figure_2.R
, 32-plot_figure_3.R
) in Jonas’s repository at https://github.com/jschoeley/openscience25/tree/main/layer2-communal/example_2-1/code. in the same way we did above. We would suggest that because these plots are final output images, instead of returning ggplot2
objects from the plot making functions, you save the plots to files and return the path to the file, like we did with the step that downloaded the data from Zenodo.